Fake-news, flash-bangs
and why statistical tests matter
Dr Bill Johnston
Main Points
Comparing instruments using paired t-tests, verses unpaired tests on daily data is inappropriate. Failing to verify assumptions, particularly that data are independent (not autocorrelated), and not considering the effect of sample size on significance levels creates illusions that differences between instruments are significant or highly significant when they are not. Using the wrong test and naïvely or bullishly disregarding test assumptions plays to tribalism not trust.
Investigators must justify the tests they use, validate that assumptions are not violated, that differences are meaningful and thereby show their conclusions are sound.
Discussion
Paired or repeated-measures t-tests are commonly used to determine the effect of an intervention by observing the same subjects before and after (e.g., 10 subjects before and after a treatment). As within-subjects variation is controlled, differences are attributable to the treatment. In contrast, un-paired or independent t‑tests compare the means of two groups of subjects, each having received one of two interventions (10 subjects that received one or no treatment vs. 10 that were treated). As variation between subjects contributes variation to the response, un-paired t-tests are less sensitive than paired tests.
Extended to a timeseries of sequential observations by different instruments (Figure 1), the paired t-test evaluates the probability that the mean of the difference between data-pairs (calculated as the target series minus the control) is zero. If the t‑statistic indicates the mean of the differences is not zero, the alternative hypothesis that the two instruments are different prevails. In this usage, significant means there is a low likelihood, typically less than 0.05, 5% or one in 20, that the mean of the difference equals zero. Should the P-value be less than 0.01, 0.001, or smaller, the difference is regarded as highly significant. Importantly, significant and highly significant are statistical terms that reflect the probability of an effect, not whether the size of an effect is meaningful.
To reiterate, paired tests compare the mean of the difference between instruments with zero, while un-paired t‑tests evaluate whether Tmax measured by each instrument is the same.
While sounding pedantic, the two tests applied to the same data result in strikingly different outcomes, with the paired test more likely to show significance. Close attention to detail and applying the right test is therefore vitally important.
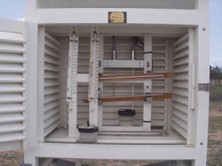
Figure 1. Inside the current 60-litre Stevenson screen at Townsville airport. At the front are dry and wet-bulb thermometers, behind are maximum (mercury) and minimum (alcohol) thermometers, held horizontally to minimise “wind-shake” which can cause them to re-set, and at the rear, which faces north, are dry and wet-bub AWS sensors. Cooled by a small patch of muslin tied by a cotton wick that dips into the water reservoir, wet-bulb depression is used to estimate relative humidity and dew point temperature. (BoM photograph).
Thermometers Vs PRT Probes
Comparisons of thermometers and PRT probes co-located in the same screen, or in different screens, rely on the air being measured each day as the test or control variable, thereby presuming that differences are attributable to instruments. However, visualize conditions in a laboratory verses those in a screen where the response medium is constantly circulating and changing throughout the day at different rates. While differences in the lab are strictly attributable, in a screen, a portion of the instrument response is due to the air being monitored. As shown in Figure 1, instruments that are not accessed each day are more conveniently located behind those that are, thereby resulting in spatial bias. The paired t-test, which apportions all variation to instruments is the wrong test under the circumstances.
Test assumptions are important
The validity of statistical tests depends on assumptions, the most important of which for paired t-tests is that differences at one time are not influenced by differences at previous times. Similarly for unpaired tests where observations within groups cannot be correlated to those previous. Although data should ideally be distributed within a bell-shaped normal-distribution envelope, normality is less important if data are random and numbers of paired observations exceed about 60. Serial dependence or autocorrelation reduces the denominator in the t-test equation, which increases the likelihood of significant outcomes (false positives) and fatally compromises the test.
Primarily caused by seasonal cycles the appropriate adjustment for daily timeseries is to deduct day-of-year averages from respective day-of-year data and conduct the right test on seasonally adjusted anomalies.
Covariables on which the response variable depends are also problematic. These includes heating of the landscape over previous days to weeks, and the effects of rainfall and evaporation that may linger for months and seasons. Removing cycles, understanding the data, using sampling strategies and P-level adjustments so outcomes are not biased may offer solutions.
Significance of differences vs. meaningful differences
A problem of using t-tests on long time series is that as numbers of data-pairs increase, the denominator in the t-test equation, which measures variation in the data, becomes increasingly small. Thus, the ratio of signal (the instrument difference) to noise (the standard error, pooled in the case of un-paired tests) increases. The t‑value consequently becomes exponentially large, the P-level declines to the millionth decimal place and the test finds trifling differences to be highly significant, when they are not meaningful. So, the significance level needs to be considered relative to the size of the effect.
For instance, a highly significant difference that is less than the uncertainty of comparing two observations (±0.6oC) could be an aberration caused by averaging beyond the precision of the experiment (i.e., averaging imprecise data to two, three or more decimal places).
The ratio of the difference to the average variation in the data [i.e., (PRTaverage minus thermometeraverage) divided by the average standard deviation], which is known as Cohens d, or the effect size, also provides a first-cut empirical measure that can be calculated from data summaries to guide subsequent analysis.
Cohens d indicates whether a difference is likely to be negligible (less than 0.2 SD units), small (>0.2), medium (>0.5) or large (<0.8), which identifies traps to avoid, particularly the trap of unduly weighting significance levels that are unimportant in the overall scheme of things.
The Townsville case study
T-tests of raw data were invalidated by autocorrelation while those involving seasonally adjusted anomalies showed no difference. Randomly sampled raw data showed significance levels depended on sample size not the difference itself, thus exposing the fallacy of using t‑tests on excessively large numbers of data-pairs. Irrespective of the tests, the effect size calculated from the data summary of 0.12 SD units is trivial and not important.
Conclusions
Using paired verse unpaired t-tests on timeseries of daily data inappropriately, not verifying assumptions, and not assessing the effect size of the outcome creates division and undermines trust. As illustrated by Townsville, it also distracts from real issues. Using the wrong test and naïvely or bullishly disregarding test assumptions plays to tribalism not trust.
A protocol is advanced whereby autocorrelation and effect size are examined at the outset. It is imperative that this be carried out before undertaking t-tests of daily temperatures measured in-parallel by different instruments.
The overarching fatal error is using invalid tests to create headlines and ruckus about thin-things that make no difference, while ignoring thick-things that would impact markedly on the global warming debate.
Two important links – find out more
First Link: The page you have just read is the basic cover story for the full paper. If you are stimulated to find out more, please link through to the full paper – a scientific Report in downloadable pdf format. This Report contains far more detail including photographs, diagrams, graphs and data and will make compelling reading for those truly interested in the issue.
Click here to download the full paper Statistical_Tests_TownsvilleCaseStudy_03June23
Second Link: This link will take you to a downloadable Excel spreadsheet containing a vast number of data points related to the Townsville Case Study and which were used in the analysis of the Full Report.
Click here to access the full data used in this post Statistical tests Townsville_DataPackage